Dimitris Kyritsis
Professor of ICT for Sustainable Manufacturing
Dimitris Kyritsis
Prof. Dr. Dimitris Kyritsis (Kiritsis) is Faculty Member at the Institute of Mechanical Engineering of the School of Engineering of EPFL, Switzerland, where he is leading a research group on ICT for Sustainable Manufacturing. His research interests are Closed Loop Lifecycle Management, Industrial Internet of Things (IIoT), Semantic Technologies and Data Analytics for Engineering Applications, Industrial Ontologies, Knowledge Graphs and Cognitive Digital Twins for industrial applications. He served as Guest Professor at the IMS Center of the University of Cincinnati, and Invited Professor at the University of Technology of Compiègne, the University of Technology of Belfort-Montbéliard and at ParisTech ENSAM Paris. Dimitris is actively involved in EU research programs in the area of Connected Factories of the Future, Zero Defect Manufacturing, Circular Manufacturing, Twin (Green and Digital) Transition etc. He has more than 250 publications. Dimitris served as Director of the Doctoral Program on Robotics, Control and Intelligent Systems of EPFL from 2019 t0 2021. From 2013 to 2019. Dimitris was the Chair of IFIP WG5.7-Advances in Production Management Systems. He is founding member of the International Society for Engineering Asset Management and co-founder of the Industrial Ontologies Foundry. Since 2019 Dimitris is member of the WEF Global Future Council on Advanced Manufacturing and Value Chains.
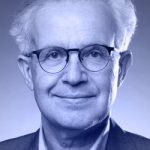
Virtual Metrology for Machining Applications
Production quality and process efficiency are the two main drivers that lead any industrial strategy. To ensure product quality, a duality historically existed between two approaches, namely batch sampling and systematic sampling. In batch sampling, the batch homogeneity is assumed, and a subset is measured, whereas, in systematic sampling, every part of the batch is measured independently. The latter approach is too expensive to be considered. Therefore, batch sampling is implemented, which, however, involves sacrifices in product quality, as the homogeneity assumption cannot always be true. Today, virtual metrology (VM) is emerging with the promise of enabling a new standard for quality control by offering a third approach that combines the benefits of the others. VM estimates product quality while preserving process efficiency. It enables continuous systematic monitoring of production in hidden time. To do so, it leverages process data and relates them to product quality through machine learning algorithms. In this work we focus on VM for milling operations, with a specific attention on the estimation of the dimensional quality of single pass milled parts. To do so, two experiments were conducted in an industrial environment. Various combinations of recorded process variables, data synchronization algorithms, dimensionality reduction algorithms, and regression algorithms were tested to maximize model accuracy. A new baseline for dimensional quality estimation in an industrial production environment is set with a mean absolute error of 14.4 μm. Besides the proof of concept, we provide directions on how to design and tune a VM algorithm for milling operations.