Serafeim Moustakidis / Eleni Lavasa
CTO / AIDEAS OU, Estonia & Athena RC, Greece
- Dr Serafeim Moustakidis is an experienced AI researcher, entrepreneur, and AI strategist. With over 15 years of experience in various AI fields, he has published over 100 scientific papers and been involved in the management and implementation of more than 30 big R&D projects. Dr Moustakidis is dedicated to developing innovative solutions to complex problems in the fields of Big Data, Data Mining, Machine Learning, Deep Learning, and Explainable AI. As the founder and Chief Technology Officer of AIDEAS OU, he is leading a team that creates cutting-edge AI solutions for healthcare, energy, and manufacturing.
-
Eleni Lavasa is a Research Assistant at the Institute for the Management of Information Systems (IMIS) of ATHENA Research Center and a Ph.D. candidate co-supervised by NOA/IAASARS and the University of Athens. She holds a B.Sc. in Physics and a M.Sc. in Space Science, Technologies & Applications. Her scientific interests mainly lie in Solar/Space Physics & the foundations of Space Weather, Machine Learning (ML), Deep Learning (DL), and explainable Artificial Intelligence (AI). She has a strong background in data analysis and in the last few years she has gained significant experience in the application of ML, DL & XAI methods in both industrial settings and in Space Weather prediction schemes. Her first publication towards her Ph.D., entitled “Assessing the predictability of Solar Energetic Particles with the use of Machine Learning techniques” was highlighted as an Editor’s Choice paper by Solar Physics. In parallel, she is involved in STEM education through the design and realization of STEM labs on Astronomy, Astrophysics & Space Science for the students of Primary & Secondary education.
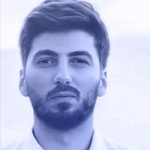
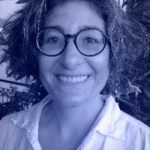
Predicting Point-Wise Measurement Accuracy of Scanning Devices using XAI-based Approaches: Towards Metrology 4.0
This presentation focuses on using explainable AI techniques to predict the accuracy of a scanning laser device. By collecting data on the geometrical properties of different calibrated objects and scanning conditions, we trained two models – a Neural Network regressor and a Support Vector Machine regressor. These models provide accurate predictions of measurement accuracy and valuable insights into how each scanning parameter contributes to the sensitivity of the scanner. We relied on explainable AI techniques to provide clear and understandable explanations for our models’ predictions, enabling metrologists to better understand and trust our results. By using these models, metrologists can optimize the scanning process by making informed decisions about scanning conditions, leading to more efficient and accurate measurements.