Daniel Campbell
Capvidia
Mr. Daniel Campbell
Mr. Daniel Campbell is the VP of MBD at Capvidia. He has over 20 years of experience in digital metrology, software design, and MBD (model-based definition). Mr. Campbell is the Chair of the QIF Working Group, a member of the Board of Directors of the Dimensional Metrology Standards Consortium, and a member of the Technology Advisory Committee for MxD Chicago.
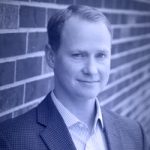
MBD in Action: Schneider Electric Use of MBD for Design and Quality
MBD (Model-Based Definition) is the process of using an annotated 3D CAD model that contains all product information data (aka. manufacturing data) to be the authoritative source downstream.
The benefits are to automate time-consuming manual tasks, reduce errors and rework through a single source of truth, and have a systematized and repeatable workflow able to produce better, faster, and cheaper.
However, for MBD to work in an industry fragmented by different CAD/CAM systems, software, and personnel, three conditions must be met: interoperability, data traceability, and semantic data.
In this presentation featuring Schneider Electric and Capvidia, we will demonstrate Schneider Electric’s approach to the use cases of MBD as we connect the digital thread across the product lifecycle in engineering, manufacturing, quality, supply chain, tolerance analysis, operations, and more.
Advanced topics to be discussed:
- Digital design in 3D including tolerance analysis.
- 3D MBD and FMEA requirements flowing to inspection planning.
- Inspection plan delivery to suppliers.
- Detailed measurement data collected from suppliers.
- Measurement data from suppliers correlated to the MBD model using QIF.
- As-built measurement data fed back into tolerance analysis for iterative improvements to design to close the digital loop.
The end result for major OEMs and enterprise end-users?
- As-designed, as-built, as-used outcomes.
- 3D digital experience.
- Seamless real-time data management.
- Interoperability of data models.
- Faster decision process, time to value, and enhanced analytics throughput.
- Improved part qualification and production readiness through quicker data access.
- Reuse of data model information and data knowledge.