Herminso Villarraga-Gómez
Carl Zeiss Industrial Metrology
Deep learning workflows for Improving scan time and image quality in 3D X-ray microscopy
In high-resolution X-ray CT, also known as 3D X-ray microscopy (XRM), long data acquisition times (in the order of hours) are required to overcome low photon counts for dense samples. Reducing the number of radiographic projections (Np) acquired for CT reconstruction, while maintaining image quality, can be effective to decrease measurement cost, depending on the reconstruction approach. With traditional filtered-back-projection based reconstruction techniques, e.g., Feldkamp-Davis-Kress (FDK) algorithm, reducing Np can cause a significant loss in image quality. This would reduce measurement accuracy and repeatability. Typical Np thresholds for a faithful 3D image reconstruction, defined by the Nyquist-Shannon sampling theorem, are in the order of two thousand projection views with modern XRM instruments. As an alternative, Deep learning (DL) based image reconstruction is an emerging technology for reducing Np further, even down to a few hundred projections, without a significant loss of image quality. DL-based algorithms for CT reconstruction improve the scan time (throughput) and image quality capabilities of XRM. This paper presents the integration of DL-based techniques into 3D XRM workflows, illustrated by several application examples (using scan data from a ZEISS Xradia 620 Versa X-ray microscope). The benefits of employing DL-based reconstruction techniques to improve 3D XRM measurement performance by reducing data acquisition time and increasing signal-to-noise ratio (enhancing image quality) are highlighted and discussed.
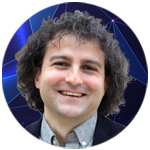
Herminso Villarraga-Gómez is an X-ray Quality Solutions Manager at ZEISS Industrial Quality Solutions. He specializes in industrial applications of X-ray computed tomography working in dimensional metrology, 3D X-ray imaging/microscopy, and nondestructive evaluation. Herminso holds a PhD in Optical Science and Engineering (University of North Carolina at Charlotte). His current interests include contributing to the industry of quality control and advancing new manufacturing technologies such as additive manufacturing and new energy/battery materials. Herminso is the author of several peer-review articles, conference papers, and a book chapter. He is a member of the American Society for Precision Engineering (ASPE), the American Society of Nondestructive Testing (ASNT), the International Societies for Optics and Photonics SPIE and OPTICA, the Society of Manufacturing Engineers (SME), and other professional associations promoting the advancement of engineering and applied physics.